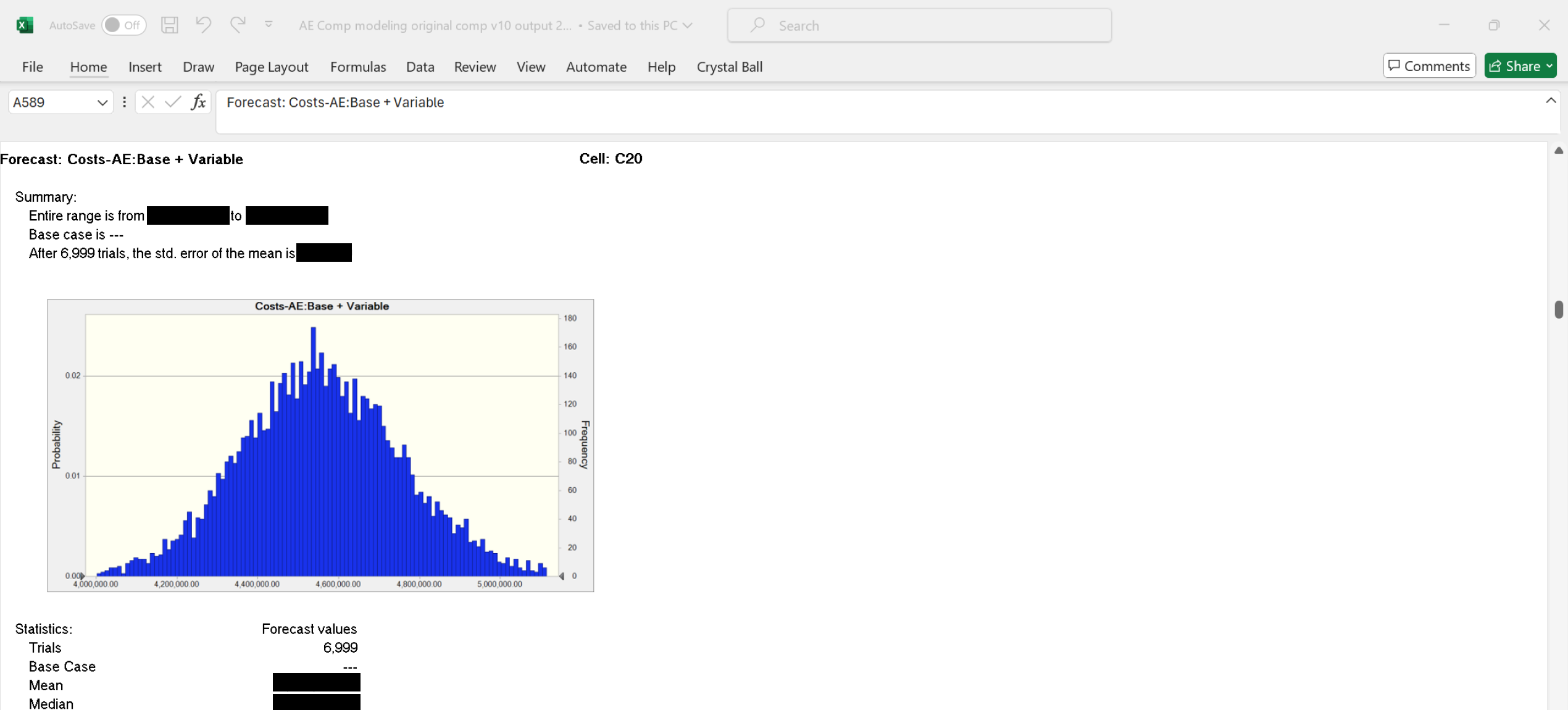
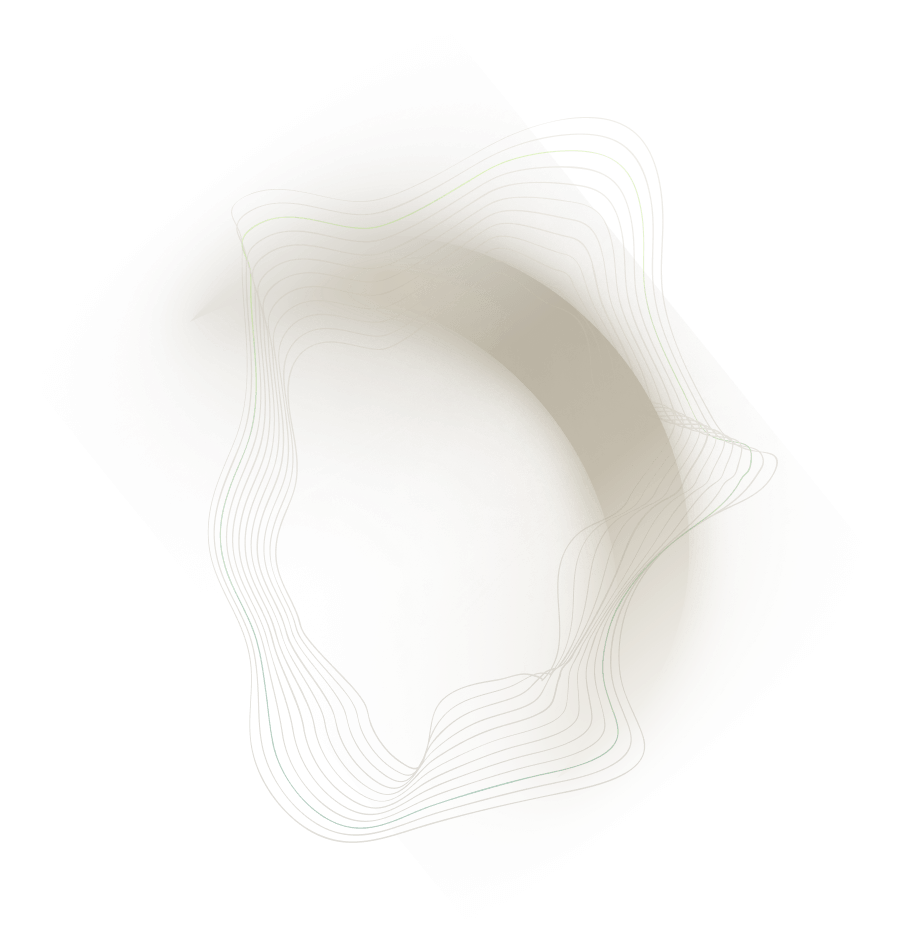
Context
Our client was a SaaS company in the US with ARR of around $120M. As part of a sales effectiveness improvement initiative, the leadership team was evaluating potentially changing the commission structure of the company. The team knew the components to change but was uncertain about the impact on the P&L due to the complexity and many dependencies of the commission structure.
For example, AE commission depended not only on the amount of ARR closed in a month, but also on the deal lengths, payment terms, amount of prior month deals, whether they closed at least one deal every month in a quarter, and so on. Additional complexities also came from the AE managers' commissions being dependent on quotas that fluctuated month-to-month depending on the size of their teams, how many of their team members were fully ramped, and a quota relief system where the quota could be lowered by a certain percent in a month if the team's collective quota was above a threshold.
Objectives
The VP of Sales Operations asked us to quantify the cost impact of their proposed changes to the commissison structure, as well as creating an Excel model that would allow his team to quantify other changes they might consider in the future.
Project delivery
We first had some sessions with the client's finance team, who was responsible for the monthly calculations of AE and manager commissions, to understand and document all the factors that determined commission payout. After analyzing all the factors, we concluded that a Monte Carlo model was the best approach to deliver what was requested.
We set up the model to mimic the new logo organization, with the same number of AEs and managers but allowing for the ability to add or reduce some. We performed historical analysis to determine the probability distribution of an AE closing a certain number of deals in a month, initially a Poisson distribution, but opted for a custom distribution based on actual numbers. We also ran a series of analyses to come up with the probability distributions of all relevant characteristics of those deals, such as deal length in months, contract MRR, payment term, etc. Using these distributions, we were able to randomly generate any number of deals, each with a specific combination of characteristics that were realistic. We then used this body of simulated deals in the model to calculate the total commissions spend in a year.
The model allowed us to not only adjust any part of the commission structure, but also modify the new logo organization structure, to project future spending. After we had set up the model, we first ran it using the current commission structure, in order to match its output to the actual commissions spend for a year. Once this calibration step is done, we changed the structure to the proposal and calculated the impact.
Outcome
The Monte Carlo model outputted the new commissions spend at multiple confidence intervals, so we were able to provide the team with worst, expected, and best case scenarios.
The model output showed the proposed changes would lead to $1.4M higher annual commissions spend, mainly driven by the lowering AE quotas. This amount was several times higher than what the team had budget for, so it prompted them to explore alternative options. Once the model was set up, the team was able to quickly check multiple alternative proposals, as well as checking the impact of adding more AE managers.
Understanding current commission structure, structuring model, determining historical probability distributions for 11 variables, verifying results, iterating outputs
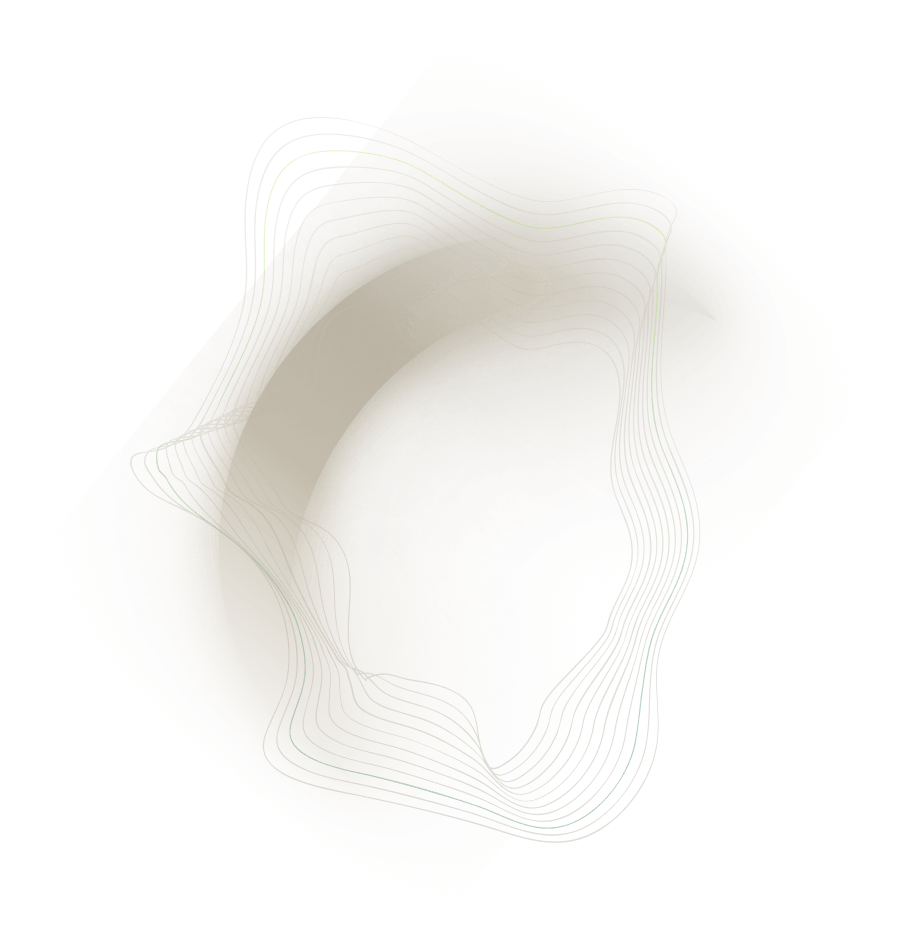
