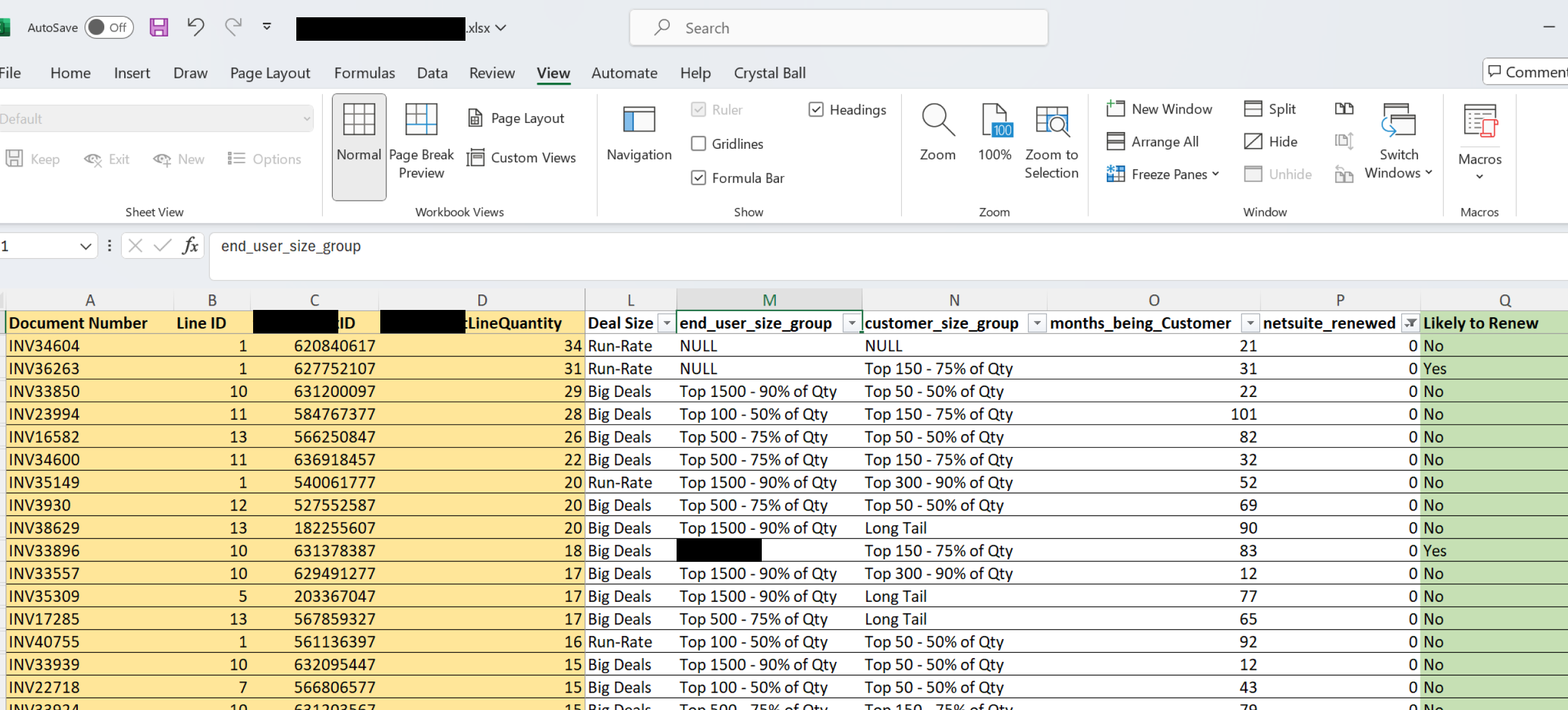
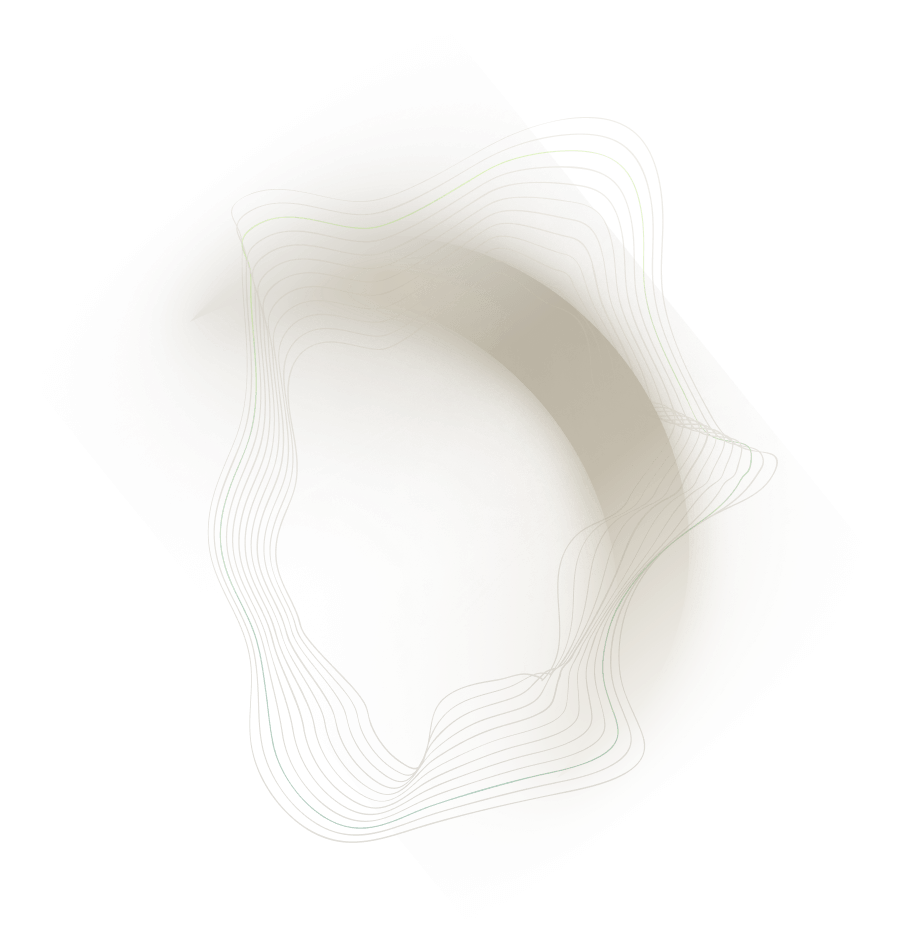
Context
Our client was a hardware technology vendor in the US with annual revenue of around $40M, and its product is used in corporate head offices around the world. While its primary business is selling hardware, a significant portion of the revenue comes from annual recurring revenue (ARR) through maintenance subscriptionsw. The renewal team is responsible for ARR renewal, a process which typically starts 6 months before contract end date.
Due to the impact of WFH on its business, the client needed to downsize, impacting both new logo and renewal teams. With a smaller team, the client needed a way to prioritize customers more likely to renew, as they no longer have the resources to pursue all customers.
Objectives
The Sales VP asked us to create a "hit list" of the customers, those that were coming up for renewal in the following 12 months and were more likely to renew their subscriptions. The renewal team would use this list to prioritize which customer to spend more effort on.
Project delivery
The overall approach we used for this analysis was to find correlations in the historical data between customer and subscription characteristics, versus renewal status. We could then use these correlations to predict likely future renewals to generate the "hit list". We noted that the level of effort of the renewal team might also have influenced past renewals besides customer and subscription characteristics, but talking with the team led us to conclude that when they had a full team in place, their process more or less gave all expiring subscriptions the same level of effort.
From the client's data warehouse, we generated a table of all historical subscriptions expiring after the pandemic, whether they were renewed or not, along with 25 subscription and customer characteristics.
We then leveraged Microsoft Azure's AutoML to run the data through multiple machine learning models, as well as a large number of different combinations of the characteristics, to determine which of them most correlated with renewal. We sanity checked the characteristics identified by the model with the VP of Sales to make sure they made sense from the business perspective.
Outcome
We found that the characteristics that most correlated with renewal were: current contract type, current contract length, customer size, customer tenure, and customer name. The first four make intuitive sense. The last one, customer name, also makes sense as some specific customers are just more likely to renew than others.
The predictive power of the model was decent, with an accuracy of 68.1% against a random chance of 50.1% in the test data, which was not present in the training data. The practical impact of this for the renewal team is that now, every 2 in 3 (67%) customers they go after would renew, whereas using their previous process, only every 1 in 2 (50%) customers would renew. This means the team can accomplish the same amount of renewal with 25% fewer staff.
The two specific output of this analysis were:
- We deployed the model to a batch inference endpoint using AutoML, which allowed us to run the future contract expiries through the model to create the "hit list" that the VP of Sales had requested
- We utilized the "hit list" and the model's accuracy statistics to assist the CFO in forecasting renewal revenue for the second half of the year
Deveoping hypotheses, investigating data, running predictive models, interpreting outputs, iterating models, generating outputs
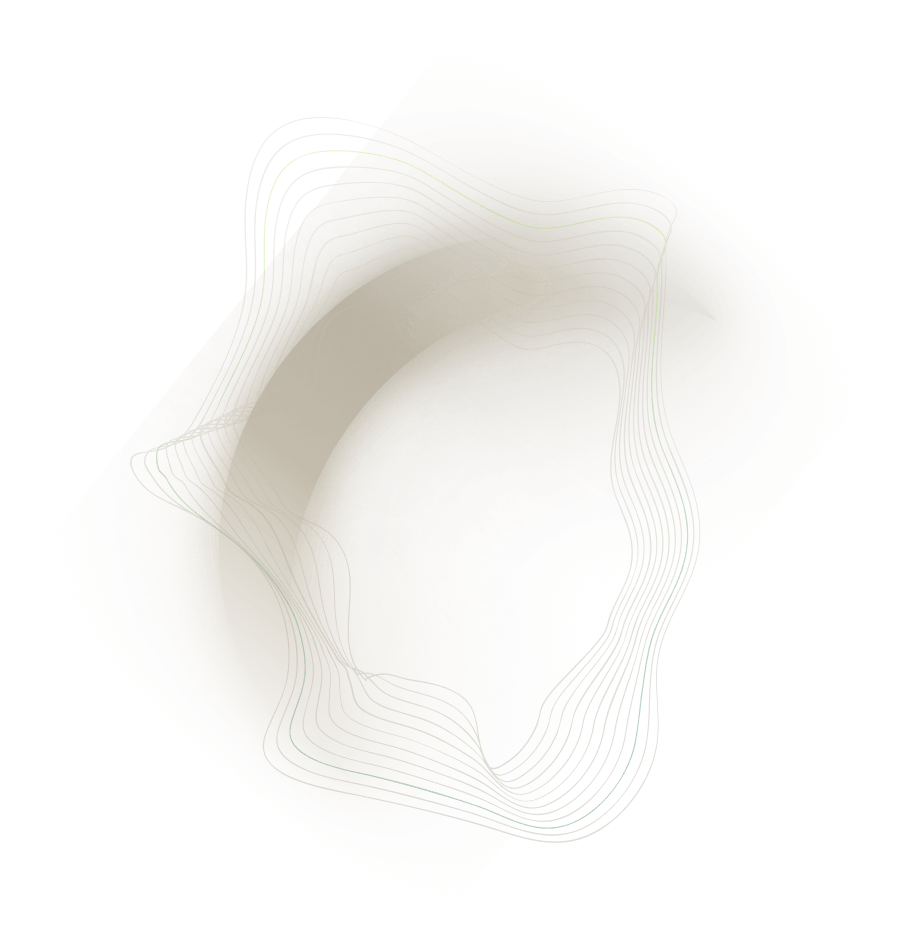
